Abstract
A smiling customer is typically considered a happy customer. An in-depth analysis of the functions of a customer’s smile is nevertheless lacking. The current paper aims to fill this research gap by presenting a video-based study on salesperson–customer interactions that uses multimodal conversation analysis as a methodological and theoretical framework. Based on a deep investigation of real-life insurance sales meetings and business-to-business role play organized at a research laboratory and a computer-based algorithm analysis of the customer’s facial expressions, the study demonstrates that a customer’s smile should not be understood as simply signaling customer satisfaction. Our findings inform sales managers and the salesforce to consider customers’ smiles as a potential signal of customer’s discomfort, which should not be pushed too far.
Keywords: emotion; negotiation; facial expressions; smile; personal selling
Introduction
A customer’s smile is often perceived as evidence of a happy customer. It is a common practice in companies to instruct personnel to service their customers with a smile to encourage positive emotions and service satisfaction (Söderlund & Rosengren, 2008). In turn, induced positive emotions have been connected to increased cooperative tactics and enhanced quality of agreements (Kopelman et al., 2006). However, an in-depth analysis of the functions of a customer’s smile is still lacking. The current study aims to fill this research gap through video-based analysis of salesperson–customer interactions. Focusing on the moments of a customer’s smile, we examined the conversational or contextual factors that trigger a customer smile and the consequences of the customer’s smile for the sales negotiation. Thus, the current study adds to our understanding of sales negotiations and customers’ emotional expressions. The results of the study inform sales managers and salesforces not to consider the seemingly positive emotional expression as a simply positive signal of customer satisfaction.
In recent years, the micro-level analysis of audio- or video-recorded salesperson–customer negotiations has attracted growing interest (e.g., De Stefani, 2018; Huma et al., 2018; Kaski et al., 2018; Mikkola & Nissi, 2020; Niemi & Vuori, 2021). The current paper adds to this research line and reports on a pilot study of customers’ smiles in salesperson–customer interactions. In addition to the video-based study of salesperson–customer interactions, the current study utilizes an experimental laboratory method, a computer-based analysis of customers’ facial expressions. The study demonstrates how the combination of a qualitative research method and laboratory measuring instruments offers a novel perspective on salesperson–customer interactions. It aims to open a debate on the possibilities of mixed methods in the study of salesperson–customer interactions, thus uncovering potential areas for its development (see also Niemi & Heinonen, 2020).
Due to its nature as a pilot study, this study should be considered an initial examination of the meaning and functions of customers’ smiles in salesperson–customer interactions. We begin our study by reviewing the research literature on customers’ smiles and smiling in more general terms, and then by describing our research setting. The section that explains the results of the research is followed by a summary and a discussion in which we consider the implications of the study for educating the future sales professionals.
Smiling: From an expression of joy to a remedy to a problematic action
To the best of our knowledge, the functions of customers’ smiles have not been the focus of prior research. However, there are studies related to customers’ smiles. Most of these discuss emotional contagion and encounter satisfaction (e.g., Barger & Grandey, 2006; Pugh, 2001). Söderlund and Rosengren (2004) examined a role-playing scenario in which a customer interacts with a hotel service employee. The study found that a smiling service employee affects the customer’s experience of joy, resulting in a higher score for customer satisfaction and often a smiling customer (see also Hennig-Thorau et al., 2006; Otterbring, 2017). Lin and Lin (2011) studied emotional contagion in service encounters. They found that employees’ inner motivation, work group mood, and service environment had a positive effect on their emotional delivery. This, in turn, had a positive influence on customers’ emotional experiences, which could result in a customer smile. As part of their study on positive emotions in clothing and accessory store interactions, Kim and Yoon (2012) examined the mediating role that the customer’s smile plays in the employee’s positive mood. They had two observers coding both the employees’ and customers’ emotions during the service interactions and conducted a post-interaction survey for the employees. The study found that employees’ display of emotions (greeting, thanking, smiling, eye contact, and pleasantness) was associated with customers’ display of emotions, and vice versa.
Clearly, much of the extant research pertaining to customers’ smiles has captured it as a result of a good service, and thus as a desired outcome. However, the customer’s smile and its function in service interactions have not been the subject of detailed analysis. In-depth qualitative research on customers’ smiles is lacking, and the current paper aims to fill this research gap. Nevertheless, there are studies connected to smiling outside the context of selling that offer interesting comparison points. Smiling is perhaps most commonly understood as a response to external stimuli of an amusing kind (Koestler, 1966, p. 28). It is often connected to laughter and the expression of joy. However, research has demonstrated that smiling and laughter may also occur in more challenging interactional contexts (for an overview of laughter as a social action, see Mulkay, 1988, p. 93–119). For example, Jefferson (1984) demonstrated that when sharing about their troubles, people may laugh, and by laughing, they claim to be ‘troubles-resistant’ in the sense of being able to take the challenges lightly. Similarly, in his study of doctor–patient interactions, Haakana (2001) showed that patients’ laughter occurs within delicate activities. Through their laughter, patients’ signal the problematic nature of the activity and seek a remedy to the problem by indicating awareness of the delicacy. These studies have focused on laughter, to which smiling is most of the time connected; however, smiling can also occur without laughter.
The study of facial expressions in social interaction has discussed their use as emotional responses. In her experimental study with university students, Chovil (1991) defined different categories for what she called discourse-oriented facial displays. These include (i) syntactic displays that emphasized particular words or clauses, or are associated with the structure of talk (e.g., initiation of topics), (ii) semantic speaker displays that conveyed information that are also expressed in words (redundant displays) or information only conveyed via the facial display (nonredundant displays), and (iii) listener comment displays (e.g., back-channel responses produced while listening to another speaker). Another study using quasi-natural interactional data found that the story recipients’ facial muscle activation reflects the valence of the storyteller’s affective stance (Voutilainen et al., 2014). When the story was happy, the story recipients’ facial muscles related to smiling were activated, but in receiving sad stories, facial muscle activation related to frowning was observed more often.
Relating to the facial displays associated with the structure of talk, earlier research has demonstrated that facial expressions can stretch the boundaries of social activities: a smile before the onset of an evaluative assessment of a given object or situation can imply that the evaluation will be positive, and a smile as a response to a neutral evaluation can signal the recipients’ humorous stance toward the evaluation (Ruusuvuori & Peräkylä, 2009). Studying turn-opening smiles in mundane conversations, Kaukomaa et al. (2013) found that a pre-beginning smile initiates a shift from a neutral or serious emotional stance to a positive or humorous stance and that such smiles persist into the utterance they are used to project. The recipients of these utterances regularly reciprocate the shift in emotional stance and produce markers of positive or humorous stance, such as laugh tokens or positive assessments. Our data offer an interesting comparison to the results of this study, as we focus on task-oriented, institutional interactions between a salesperson and a customer. As we will demonstrate, customers’ smile to initiate an utterance, but the function of these early smiles resembles more the functions of smiles observed by Haakana (2001) and signal a potentially delicate activity to follow.
Methodology and data
As a methodological and theoretical framework for this study, we draw on multimodal conversation analysis (e.g., Goodwin, 2000; Mondada, 2006, 2009; Mortensen, 2012). It is based on ethnomethodological conversation analysis (e.g., Hutchby & Wooffitt, 2008; Sidnell & Stivers, 2012), and it analyses how the participants temporally and sequentially co-construct the ongoing interaction and build a mutual understanding through different types of turn-formulations and actions (see Schegloff, 2007). Conversation analysis has been widely applied in different types of institutional settings, for instance, in workplace interactions and customer service situations (see e.g. Antaki, 2011; Heritage & Clayman, 2011). Multimodal conversation analysis does not focus solely on verbal interaction but emphasizes the meaning of multiple modalities of interaction, especially embodied practices (such as gazes, postures, gestures, etc.). The relevant point of view concerning our research setting is the role of gazes, facial expressions, gestures, and postures. The meaning of embodied practices has been highlighted as relevant in the field of multimodal conversation analysis, including institutional interaction and its practices (e.g., Heath & Luff, 2011; Mortensen, 2012).
In the current study, the customers’ facial expressions were automatically analyzed using an AFFDEX algorithm. This analytical tool is included in the iMotions software (www.imotions.com), and the analysis is built on the Facial Action Coding System developed by Paul Ekman and Wallace Friesen (Rosenberg, 2005). This coding system is based on 46 distinct facial movements, such as nose wrinkle, cheek raise, and lip corner depression. Drawing on the fine-tuned coding system, the algorithm estimates probabilities for different emotions based on the combination of informant’s facial movements. For example, the likelihood for the emotion of “joy” increases if lip corners and cheeks are raised, and lowers if eyebrows are raised or frowned. Based on the laboratory data, a graph was automatically generated in the iMotions software. In the graphs, the likelihood of a given emotion (as well as facial expressions such as a smile) is displayed as a vertical peak on a horizontally evolving line (Picture 1). The higher the peak, the more certain the algorithm is that a given emotion is expressed.
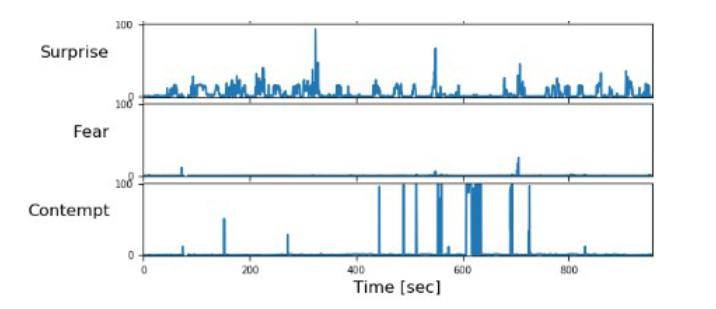
A validation study (Stöckli et al., 2018) confirmed that AFFDEX can recognize facial expressions and emotions based on them. However, the study concluded that the algorithm is most accurate when it is applied to static pictures instead of moving pictures, such as live video. Thus, when applying AFFDEX in the study of interactional data, attention must be paid to the possibility of false recognition or lack of recognition of emotions. In practice, the results given by the algorithm need to be confirmed by human observation, which is the starting point of this research setting. In analyzing specific key moments in sales interaction, the combination of laboratory data and detailed interaction analysis may offer a more nuanced and deeper understanding of the research topic.
As data, this study draws on (1) video-recordings made at the Emotion & Interaction Lab at Haaga-Helia University of Applied Sciences, as well as (2) video-recording data captured earlier outside the Lab, and later imported to the Lab for analysis. The first dataset that was video recorded in the Lab was an organized role-play between a salesperson and a customer, and the salesperson represented a fictional company operating within staffing services, while the customer represented a company in the manufacturing industry. Thus, the context of the roleplay was business-to-business sales interactions. The role-play took place in 2019.
The second dataset video-recorded outside the Lab consists of a real-life insurance sales negotiation organized at the premises of the insurance company. The data were collected in 2016 as part of the Digitalization in Customer Interaction (DICIA) research project, funded by Business Finland as well as partnering companies and universities (Aalto University, Haaga-Helia University of Applied Sciences, and University of Helsinki).
As for the first dataset collected in the Emotion & Interaction Lab, it was easier to apply the facial expression analysis tools. The sales interaction was organized as a role-play that we could manipulate in a way that a USB video camera was facing the customer directly and closely (Picture 1). Thus, the algorithm had a clear and close view of the customer’s face. In Picture 1, a face box generated by AFFDEX is clearly visible on the customer’s face, as well as the 34 points of interest whose relative movement is constantly analyzed to observe probabilities for different emotions. As shown in the picture, most of these points are located on the lips and around the eyes. On the pictures that we display, we have added white boxes to cover the research participants eyes.
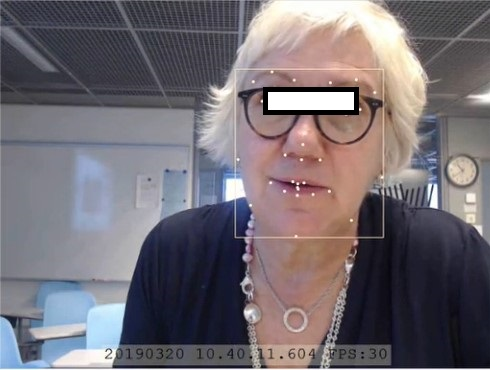
By contrast, the real-life insurance meeting (second dataset) was video-recorded by two stand-alone video cameras, both capturing the overall picture of the sales negotiation (Picture 3), but from opposite directions. Thus, we did not have a camera dedicated to capturing the customer’s facial expressions. As a result, the customer’s face is more distant from the camera, and there is roughly a 30-degree angle between the camera and the customer’s face. Thus, the algorithm was not able to analyze the customer’s facial expressions all the time. At best, we received approximately 40% of the video recordings across the period analyzed by the AFFDEX algorithm, with the lowest being roughly 30%. In Picture 3, the face box generated by AFFDEX is much smaller than the one we saw in Picture 2.
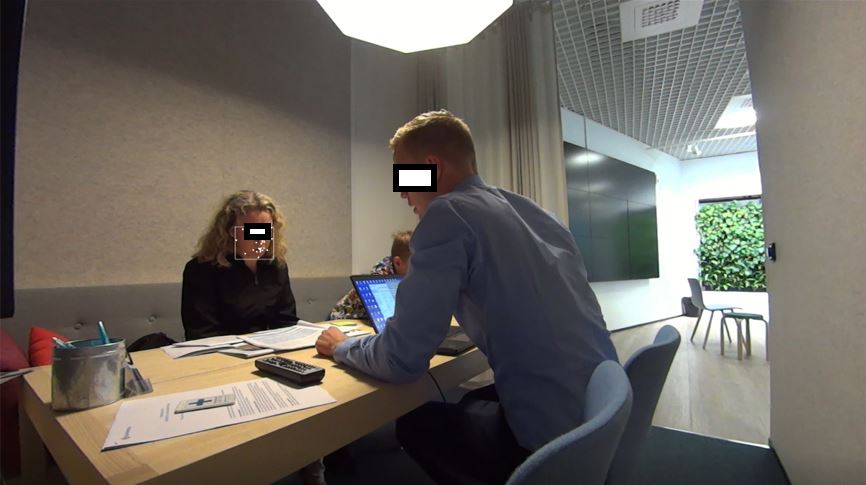
The facial expression analysis for this meeting is based on a video-recording that was later imported to the computer with the iMotions software at the Interaction & Emotion Lab. According to the iMotions software instructions, we inserted a designated area for the face to be analyzed in the recording. This area included the customer’s face and some room for movement (e.g., leaning back or forward in her chair; see Picture 4).
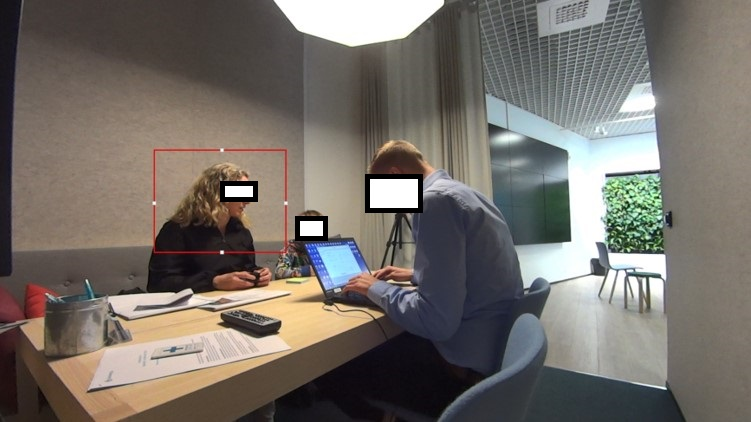
After defining the area to be analyzed, we applied the AFFDEX algorithm to analyze the customer’s facial expressions. However, the algorithm was not able to analyze all instances of the customer’s smiles. This illustrates the downside of using data that were recorded without a specific intention and thus proper arrangements (e.g., having a video camera directly in front of the target person) to analyze facial expressions.
Our two-part data allowed interesting possibilities for observations of the usability of the facial expression analysis tools. Given that we had data collected at the lab and data collected outside the lab, we could compare the two and their usability and reliability as data to be analyzed. Altogether, the analysis reported in this paper is based on two video recordings, which does not allow us to generalize. However, we were able to observe the contexts for customers’ smiles and test the usability of facial expression tools as part of a qualitative analysis.
Analyzing customers’ smiles in sales interactions
We begin our analysis with a seemingly simple case of a customer’s reactive smile. That is, the customer smiles in response to something that the salesperson said. Although it is possible (and evidenced in our data) that the customer would smile as a reaction to something that he or she found amusing, this is not the case in our example. Instead, through her smile, the customer oriented to the implications of the salesperson’s utterance and marked them as delicate or as a potential reason for embarrassment.
Our first example originated from a real-life insurance sales encounter organized at the facilities of the insurance company. The customer arrives at the insurance company office with her son, who is approximately seven years old. Given that she is accompanied by her son, she needs to attend to him while negotiating with the insurance company salesperson. Thus, she requests a quick discussion, and the salesperson refers to this wish at the beginning of our extract. In our examples, SP denotes the salesperson, while C stands for the (potential) customer. The transcription symbols are explained in Appendix 1.
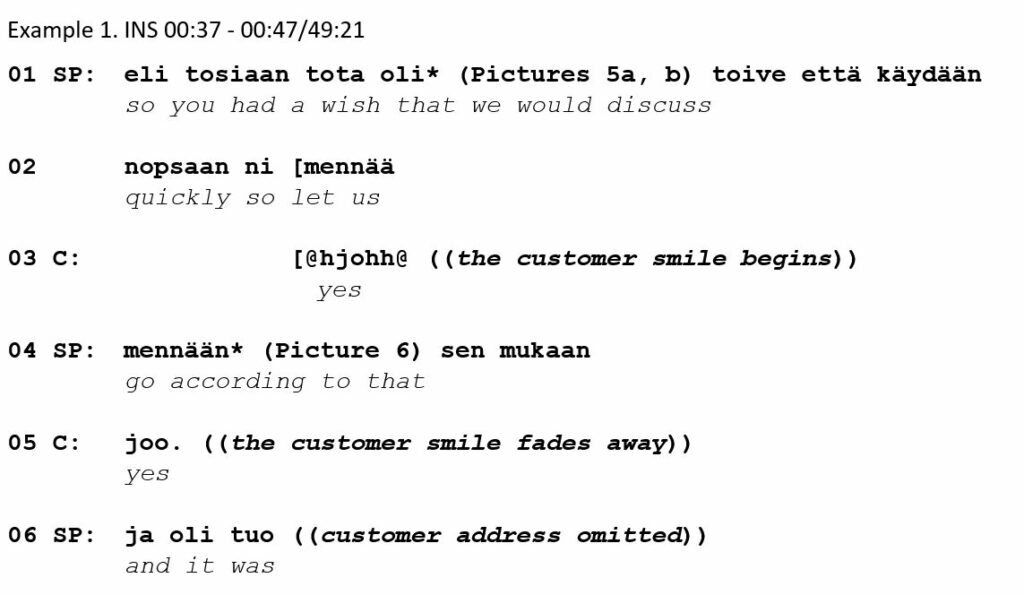

The salesperson commences the business talk by referring to the wishes made by the customer. As he begins his utterance, he gazes the computer on the table in front of him. However, he raises his gaze from the computer screen and meets the customer’s eyes when he starts to pronounce the word toive, ‘wish’ (line 1; Pictures 5a and 5b). Both parties have a neutral face.
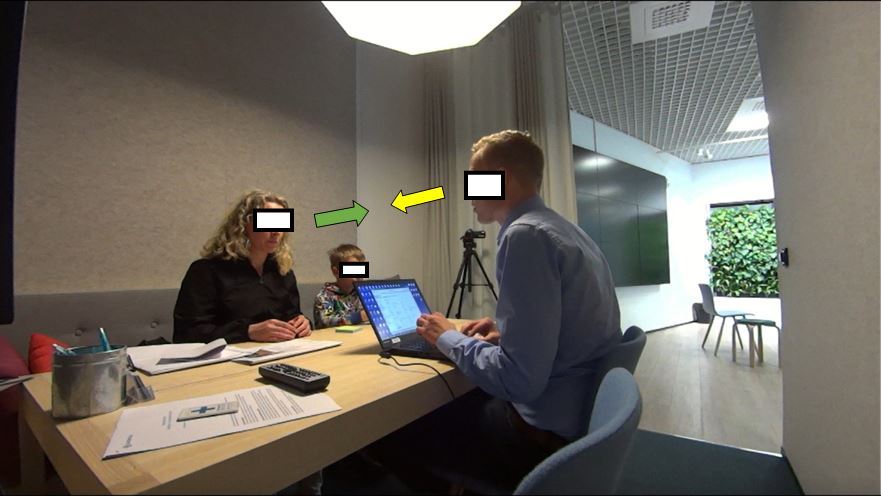
Eli tosiaan oli* ‘So you had a*’ (line 1; the asterisk marks the exact point of time when the still shot was taken)
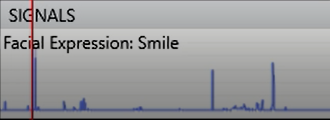
While maintaining the mutual gaze, the salesperson begins to smile (during the word nopsaan, ‘quickly’, line 2), and the customer reciprocates the smile shortly thereafter. Therefore, there is an element of emotional contagion: First, the salesperson smiles, and the smile is reciprocated by the customer. However, the customer not only smiles, but she also produces an acknowledgement token joo, ‘yes’, with a smiley voice and audible outbreath (line 3). At the same time, she withdraws from the mutual gaze, closes her eyes, and looks down (Pictures 6a and 6b). For a moment, the salesperson still looks at the customer, but shortly, he also looks down and toward the computer screen, as seen in picture 6a.
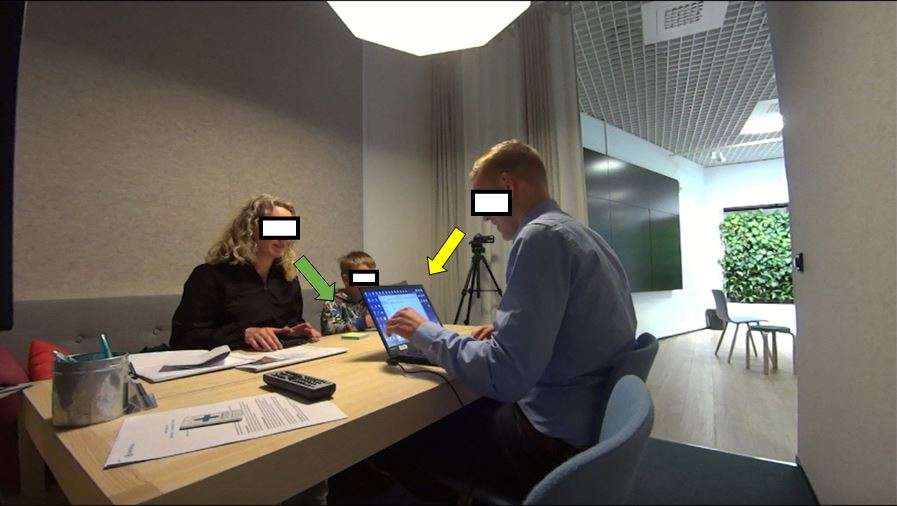
nii mennään* sen mukaan, ‘so let us* go according to that’ (line 4)
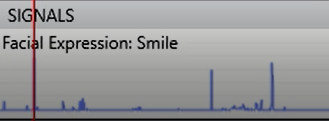
It is obvious that the salesperson did not say something clearly amusing that could have induced a mutual smile. Rather, the smiles mark a potentially delicate moment during which the customer’s wish that set organizational guidelines for the insurance negotiation is spotlighted. We argue that the customer’s nonverbal actions (first to withdraw from the mutual gaze, the closing of eyes, looking down) add to the interpretation that she marks a point of possible embarrassment. This interpretation is further supported by the customer’s somewhat subdued smile as well as the audible outbreath when she produces the acknowledgment token joo, ‘yes’, adding a token of laughter to her turn. An explanation for the embarrassment could be found from the fact that the customer, with her wish, potentially restricts the salesperson, who might have to adjust his behavior due to the customer’s wish.
As the salesperson initiates the preparatory steps for the insurance discussion to begin (checking that he has the correct contact details of the customer), the smiles slowly fade away. We observe that besides the customer’s audible outbreath when producing joo, ‘yes’, there is no laughter connected to this brief sequence of mutual smiles. This also hints at the interpretation that nothing amusing or humorous was happening. Overall, Example 1 demonstrates a common occurrence of a reactive customer’s smile in our data, one that is connected to a potentially delicate moment in the business negotiation (cf. Haakana, 2001). However, our data also provide examples of a customer’s smile as a reaction to an amusing remark by the salesperson.
Next, we shift our attention from customers’ reactive smiles to another type of customers’ smiles. The smiles we next discuss resemble the one that Chovil (1991) called syntactic facial displays, as the smile occurs on the customer’s face before the initiation of an utterance. Such usage of a smile may propose a shift from a neutral emotional stance to a positive or humorous stance, as has been noted in the study of mundane conversations (Kaukomaa et al., 2013).
Our second example originates from the role-play interaction. We join this interaction in its early moments. The salesperson has just asked how well the customer was informed about an earlier discussion between the salesperson and the production manager of the customer’s organization (en sitte tiedä kuinka paljon oot kuullut tästä meidän aikasemmasta keskustelusta, ‘I don’t know how much you have heard about our earlier discussion’; data not shown). The customer responds that she is not informed at all (en oo kuullu kyllä mitään, ‘I have heard nothing at all’) and added that she is quite busy, thereby implying a request for the salesperson to promptly proceed with the business at hand. The salesperson continues from this point on at line 1 in Example 2.
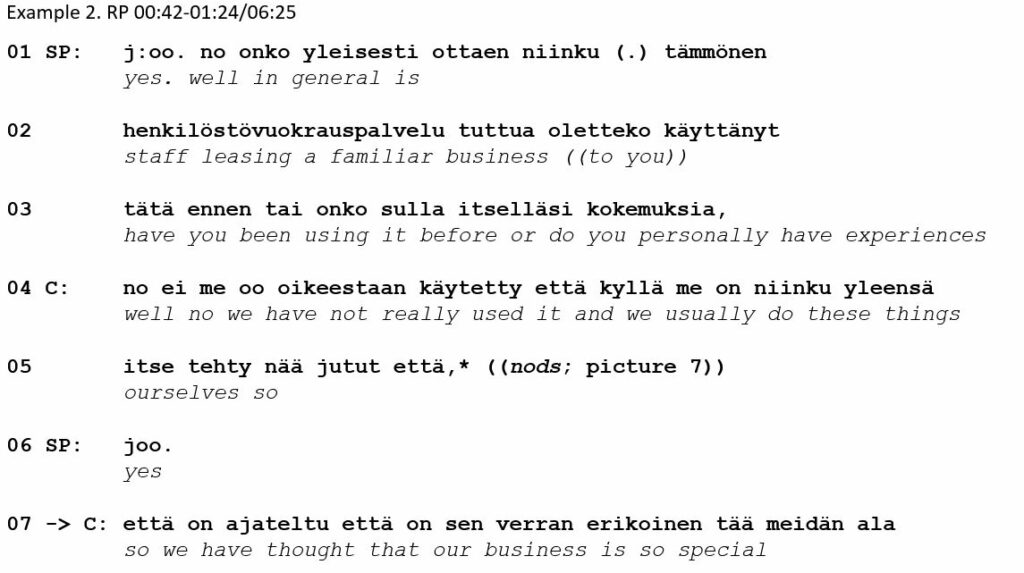
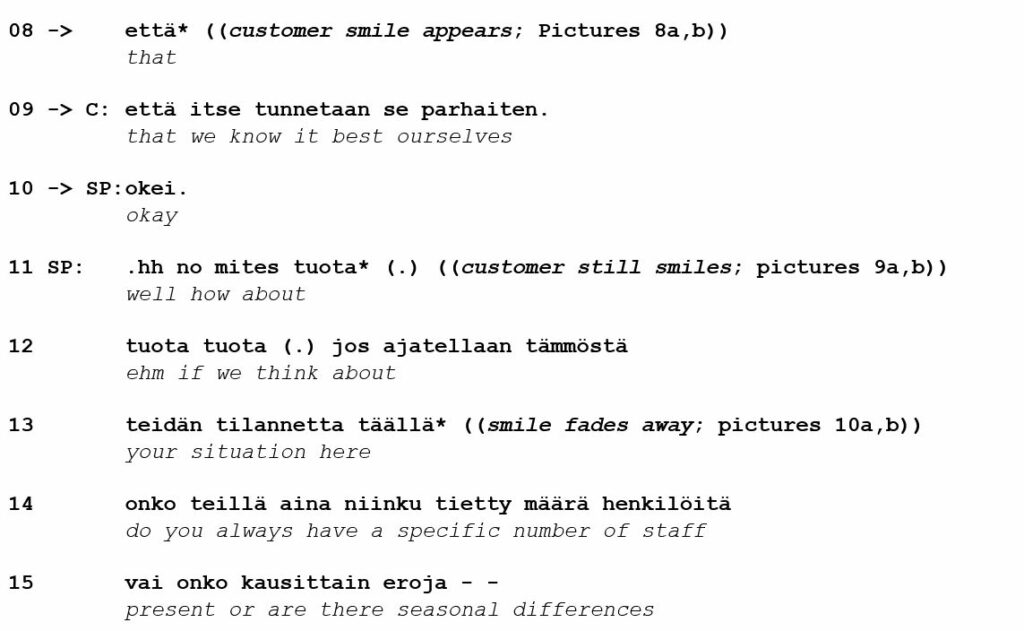
Responding to the salesperson’s question (lines 1–3), the customer declines her company’s prior experiences in using rented staff (lines 4–5). While doing this, she has a neutral face (see Picture 7).
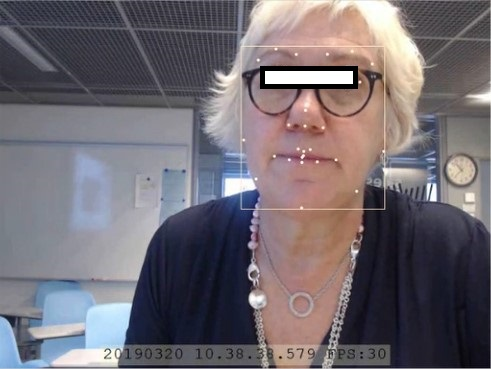
itse tehty nää jutut että* ‘We have done these things ourselves so*’ (line 5; as in Example 1, the asterisk * marks the exact point in which the still shot was taken)
However, when the customer then accounts for the organizational lack of experience, and just before providing the crux of her argumentation (line 9), she begins to smile at line 8 (Picture 8a; Picture 8b shows the graph produced by the AFFDEX algorithm).
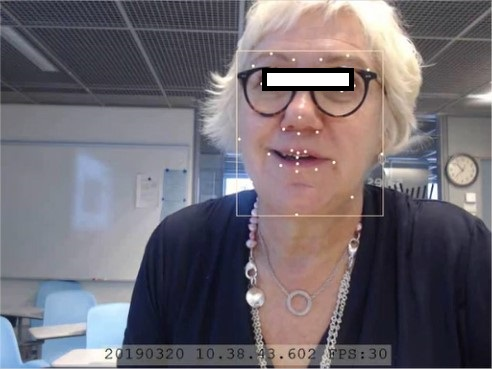
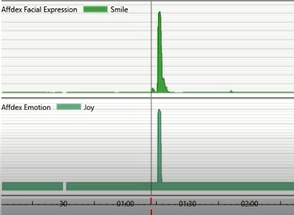
sev verran erikoinen tää meidän ala että* ‘our field is so special that’* (lines 7–8)
It is evident to us that this smile is not an emotional reaction to something that the salesperson said or something that has just happened. Rather, the smile sets up an interpretational frame for what the customer says next. The forward-looking feature of the smile is evidenced by the fact that, just before the initiation of her smile, she produces the conjunction että ‘that’, projecting a follow-up (Koivisto et al., 2011). The projected follow-up turns out to be a self-appraisal that evaluates the customer organization as the best-informed actor in their business field (line 9). Although self-appraisals in conversation are generally dispreferred, here this might not be a controversial statement to make, as arguably any organization is expected to possess the best information on its own business. However, considering the customer’s current interlocutor, a salesperson from a staffing agency, the customer’s statement might be interpreted as opposing the relevance of the service that the salesperson offers: rented staff is not used, because the customer company has the best personal resources for its own needs. Therefore, there is potential for social discomfort, which can be remedied with a smile (see Haakana, 2001; Jefferson, 1984).
Studying the data graph from the automated recognition of facial expressions, we observe that in Pictures 8a and 8b, the AFFDEX algorithm does not yet recognize a likelihood for the facial expression “Smile.” In Picture 8b, the bar that moves in time from left to right and indicates a specific moment is located before the clear peak in the graph. This might be because the customer’s smile is still somewhat subdued, with cheeks in their neutral position (unraised), but for us as human observers, it is clear in Picture 8a that the customer is already smiling. Importantly, the customer prolongs her smile from this point on to the next stages of the conversation. In this way, she pursues an emotional coherency from a sequence of interaction to the next one. However, we also note that there is no laughter connected to the customer’s smile, and that the salesperson received the customer’s self-complimenting turn neutrally with ‘okay’ (line 10). Then, the salesperson initiates a new line of action (no mites tuota, ‘well how about’, line 11). Thus, the salesperson does not reciprocate the “lighter” emotional stance potentially proposed by the customer but instead continued business as usual. By doing this, the salesperson did not attend to the critical stance expressed by the customer, according to which the salesperson’s service might not be needed.
While the salesperson continues building a new line of action (no mites tuota, ‘well how about’, line 11), the customer still smiles, as can be observed in Picture 9a.
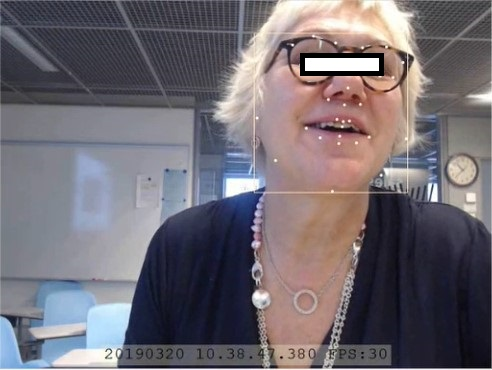
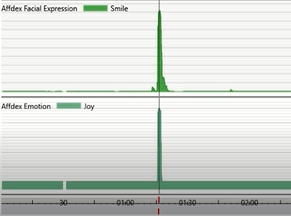
no mites tuota* ‘well how about’* (line 11)
We conclude that even though a new sequence of interaction has been initiated and verbally marked as initiated (see the particle no, roughly translatable as ‘well’, and the enclitic particle -s in mites, marking the beginning of a new but agenda-related sequence; Raevaara, 2006), the customer’s facial expression creates continuation between the already finished sequence (lines 1–10) and the new sequence (beginning from line 11). In other words, the customer’s facial expression stretches the boundaries of a social action (Peräkylä & Ruusuvuori, 2006). As Picture 9b displays, the algorithm now agrees that the customer indeed is smiling, as indicated by the high estimation of likelihood for the facial expression of smile. We observe that in Picture 9a, unlike in Picture 8a, the customer’s cheeks are raised and her eyes are narrowed.
From this point on, the customer’s smile begins to fade away, and she has a neutral face in line 13 of Example 2, when the salesperson refers to the situation at the customer’s organization (Picture 10a, b).
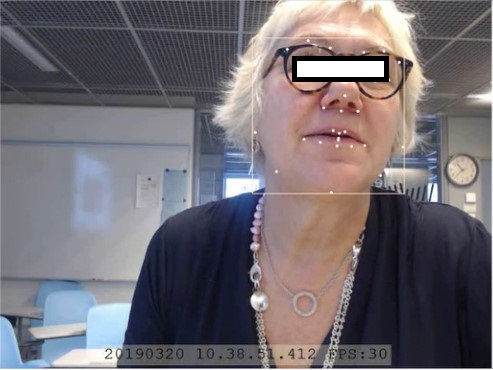
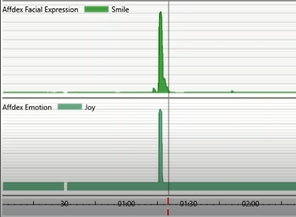
teidän tilannetta täällä* ’your situation here’* (line 13)
In summary, Example 2 demonstrates a case in which a customer smiles not as a reaction to something that was said or occurred earlier but rather before he or she initiates a new line of action. Thus, the customer’s smile set up an emotional frame in which the upcoming verbal action could be interpreted more lightly. In our example, the action that was prefaced with a smile was volunteering an account for a declining answer to a salesperson’s request for information. Importantly, the customer smiled throughout the production of the central argument of her account and after the account was received and a new line of action initiated by the salesperson. This created and encouraged emotional continuance, which extended over the boundaries of a sequence and action. However, the salesperson immediately initiated a return to a neutral emotional stance, as already observed. Thus, even though the customer produced her turn with a smile, the salesperson arguably demonstrated his understanding that the customer’s line of action was not something that he should be expanding on, given his agenda as a salesperson.
Summary and discussion
In this paper, we have discussed the functions of a customer’s smile in sales interactions. We started by observing the lack of video-based research on customers’ smiles. Extant research has considered a customer’s smile as the desired outcome after a sales negotiation, reflecting the good service that the customer has acquired. To the best of our knowledge, this paper is the first to discuss the functions of customer’s smiles in more detail, and during sales negotiations.
The current study challenges the view that a customer’s smile is simply a positive outcome from the salesperson’s perspective. In fact, it would be dangerous for the salesperson to blindly assume a positive emotional stance from the smiling customer, as the customer’s smile clearly serves more delicate functions. We discussed the customer smile as a marker of the customer’s possible embarrassment (Example 1), and as a pre-beginning element that occurs before the customer’s utterance and thus works as an interpretational frame. In this usage, the smile may imply a socially problematic action, such as presenting a challenge to the relevance of the salesperson’s service to the customer organization (Example 2). Taking these results into consideration, we advise that the sales managers and educators discuss customer’s seemingly positive emotional expression via smiling also as a potential marker of a dangerous path: it could be signaling the customer’s discomfort, which should not be pushed too far.
We applied a computer-based algorithm to analyze the customer’s facial expressions. We found that the algorithm was reasonably reliable in its observations of customer smiles, even for data that were gathered outside the Interaction & Emotion Lab and was not designed to be analyzed by the AFFDEX algorithm. We were able to confirm and focus on instances of customer smiles initially found by the algorithm. Occasionally, we were somewhat confused by the exact timing of the customer’s smile defined by the algorithm. As for us as human observers, there seemed to be signs of customer smile even before a high certainty from the algorithm (see pictures 8a and 8b). However, sometimes, we were not as sure about the customer’s smile as the algorithm seemed to be. Overall, we found that the algorithm provided us with a solid comparison point for our own observations for qualitative analysis. In the future, researchers should consider whether it is better to arrange optimal conditions for the algorithm to observe facial expressions, or whether it is better to strive for more natural conditions and have the video cameras a bit further away from the participants.
In general, this study strengthens our understanding that laboratory research on social interactions should be combined with human observation and qualitative research methods (see also Niemi & Heinonen, 2019). The laboratory devices provided results that pointed us to notice different emotions in the participants. Furthermore, by combining, for example, conversation analysis and the sequential analysis of interactions with laboratory devices, we acquire a better understanding of why emotions occur and their interactional consequences.
As our data are rather limited, further studies should extend our observations. Here, we chose not to discuss some usages of customer’s smiles that were instanced only once or twice in our data. A study with a larger dataset could thus discuss the functions of customers’ smiles more broadly. Further research could systematically observe the potential occurrence of emotional contagion: Does the customer’s smile induce a smile from the salesperson, and vice versa. Such a study could address the implications of such contagious smiles and, perhaps even more interestingly, the implications of the lack of emotional contagion for sales success. Here, we could not do this, as our data collected at Interaction & Emotion Lab did not include the video picture of the salesperson’s face. For this reason, a systematic analysis of the synchronization of participants’ facial expressions was not included in the analysis. However, this could be an interesting topic for further research.
Finally, we would like to encourage the use of role-plays in laboratory settings, such as Interaction & Emotion Lab, as a teaching method for sales students. It seems evident to us that, for example, automated analysis of facial expressions could give important hints on customer’s emotions for students who practice their sales skills. The feedback can be received live as a role-play between a salesperson and a customer is conducted. In this way, the students learn how to modify and control their behavior in a way that pays close attention to the customers’ emotional experiences.
References
Antaki, C. (Ed.) (2011). Applied conversation analysis. Intervention and change in institutional talk. Palgrave MacMillan.
Barger, P., & Grandey, A. (2006). Service with a smile and encounter satisfaction: Emotional contagion and appraisal mechanisms. Academy of Management Journal, 49(6), 1229–1238. https://doi.org/10.5465/amj.2006.23478695
Chovil, N. (1991). Discourse-oriented facial displays in conversation. Research on Language and Social Interaction, 25(1), 163–194.
Clift, R. (2016). Conversation analysis. Cambridge University Press. https://doi.org/10.1017/9781139022767
Goodwin, C. (2000). Action and embodiment within situated human interaction. Journal of Pragmatics, 32(10), 1489–1522. https://doi.org/10.1016/S0378-2166(99)00096-X
Haakana, M. (2001). Laughter as a patient’s resource: Dealing with delicate aspects of medical interaction. Text & Talk, 21(1-2), 187–219. https://doi.org/10.1515/text.1.21.1-2.187
Heath, C., & Luff, P. (2011). Gesture and institutional interaction. In J. Streeck, C. Goodwin, & C. LeBaron (Eds.) Embodied interaction: Language and body in the material world (pp. 276–288). Cambridge University Press.
Hennig-Thurau, T., Groth, M., Paul, M., & Gremler, D.D. (2006). Are all smiles created equal? How emotional contagion and emotional labor affect service relationships. Journal of Marketing, 70(3), 58–73. https://doi.org/10.1509/jmkg.70.3.058
Heritage, J., & Clayman, S. (2011). Talk in action: Interactions, identities, and institutions. John Wiley & Sons.
Huma, B., Stokoe, E., & Sikveland, R. (2018). Persuasive conduct: Alignment and resistance in prospecting ‘cold’ calls. Journal of Language and Social Psychology, 38(1), 33–60. https://doi.org/10.1177/0261927X18783474
Hutchby, I., & Wooffitt, R. (2008). Conversation analysis. Polity.
Jefferson, G. (1984). On the organization of laughter in talk about troubles. In J. M. Atkinson & J. Heritage (Eds.), Structures of social action: Studies in conversation analysis (pp. 346–369). Cambridge University Press.
Kaski, T., Niemi, J., & Pullins, E. (2018). Rapport-building in authentic B2B sales interaction. Industrial Marketing Management, 69, 235–252. https://doi.org/10.1016/j.indmarman.2017.08.019
Kaukomaa, T., Peräkylä, A., & Ruusuvuori, J. (2013). Turn-opening smiles: Facial expression constructing emotional transition in conversation. Journal of Pragmatics, 55, 21–42. https://doi.org/10.1016/j.pragma.2013.05.006
Kim, E., & Yoon, D. J. (2012). Why does service with a smile make employees happy? A social interaction model. Journal of Applied Psychology, 97(5), 1059–1067. https://doi.org/10.1037/a0029327
Koestler, A. (1966 [1964]). The act of creation. Pan Books.
Koivisto, A., Laury, R., & Seppänen, E.-L. (2011). Syntactic and actional characteristics of Finnish että-clauses. In R. Laury & R. Suzuki (Eds.), Subordination in conversation: A cross-linguistic perspective (pp. 69–102). John Benjamins.
Kopelman, S., Rosette, A. S., & Thompson, L. (2006). The three faces of Eve: Strategic displays of positive, negative, and neutral emotions in negotiations. Organizational Behavior and Human Decision Processes, 99, 81–101. https://doi.org/10.1016/j.obhdp.2005.08.003
Lin, J. C., & Lin, C. (2011). What makes service employees and customers smile: Antecedents and consequences of the employees’ affective delivery in the service encounter. Journal of Service Management, 22(2), 183–201. https://doi.org/10.1108/09564231111124217
Mikkola, P., & Nissi, R. (2020). Myyntityötä ja osallistumisen säätelyä. Tapaustutkimus taiteilijan ohjaamasta harjoituksesta henkilöstökoulutuksessa. In H. Paulasto & S. Pöyhönen (Eds.), Kieli ja taide – soveltavan kielentutkimuksen ja taiteen risteämiä. Language and the arts – Creative inquiry in applied linguistics (pp. 163–191). AFinLA-e. https://doi.org/10.30660/afinla.84538
Mondada, L. (2006). Participants’ online analysis and multimodal practices: projecting the end of the turn and the closing of the sequence. Discourse studies, 8(1), 117–129. https://doi.org/10.1177/1461445606059561
Mondada, L. (2009). Emergent focused interactions in public places: A systematic analysis of the multimodal achievement of a common interactional space. Journal of pragmatics, 41(10), 1977–1997. https://doi.org/10.1016/j.pragma.2008.09.019
Mortensen, K. (2012). Conversation analysis and multimodality. The encyclopedia of applied linguistics. Wiley.
Mulkay, M. (1988). On humor. Its nature and its place in modern society. Blackwell.
Niemi, J., & Heinonen, P. (2019). Kysymys-vastausjaksot myyjän ja asiakkaan etäneuvottelussa: keskustelunanalyysin integrointi laboratoriotutkimukseen [English abstract Question–Answer sequences in salesperson–customer interaction: Integrating conversation analysis to laboratory research]. In S. Grasz, T. Keisanen, F. Oloff, M. Rauniomaa, I. Rautiainen, & M. Siromaa (Eds.), Menetelmällisiä käänteitä soveltavassa kielentutkimuksessa – Methodological turns in applied language studies (pp. 215–241). AFinLA-e. https://doi.org/10.30661/afinlavk.89305
Niemi, J., & Vuori, J. (Eds.). (2021). Myyntityö vuorovaikutuksena [Selling as interaction]. Vastapaino.
Otterbring, T. (2017). Smile for a while: The effect of employee-displayed smiling on the customer affect and satisfaction. Journal of Service Management, 28(2), 284–304. https://doi.org/10.1108/JOSM-11-2015-0372
Peräkylä, A., & Ruusuvuori, J. (2006). Facial expression in an assessment. In H. Knoblauch, B. Schnettler, J. Raab & H.-G. Soeffner (Eds.), Video analysis: Methodology and methods (pp. 127–142). Peter Lang.
Pugh, S. D. (2001). Service with a smile: Emotional contagion in the service encounter. Academy of Management Journal, 44(5), 1018–1027.
Raevaara, L., 2006. Kysymykset virkailijan työkaluna [Questions as a clerk’s tool]. In M.-L. Sorjonen & L. Raevaara (Eds.), Arjen asiointia: Keskusteluja Kelan tiskin äärellä [Everyday errands: Conversations in the Finnish Social Insurance Office] (pp. 86–116). Finnish Literature Society.
Rosenberg, E. L. (2005). Introduction: The study of spontaneous facial expressions in psychology. In P. Ekman & E. L. Rosenberg (Eds.), What the face reveals: Basic and applied studies of spontaneous expression using the Facial Action Coding System (FACS) (2nd edition, pp. 3–18). Oxford University Press.
Ruusuvuori, J., & Peräkylä, A. (2009). Facial and verbal expressions in assessing stories and topics. Research on Language & Social Interaction, 42(4), 377–394. https://doi.org/10.1080/08351810903296499
De Stefani, E. (2018). Institutional invitations to a meeting: Cold calls to bank clients. Journal of Pragmatics, 125, 180–199. https://doi.org/10.1016/j.pragma.2017.06.011
Stivers, T., & Sidnell, J. (Eds.). (2012). The handbook of conversation analysis. John Wiley & Sons.
Stöckli, S., Schulte-Mecklenbeck, M., Borer, S., & Samson, A. C. (2018). Facial expression analysis with AFFDEX and FACET: A validation study. Behavior Research Methods, 50, 1446–1460. https://doi.org/10.3758/s13428-017-0996-1
Söderlund, M., & Rosengren, S. (2004). Dismantling “positive affect” and its effects on customer satisfaction: An empirical examination of customer joy in service encounter. Journal of Customer Satisfaction, Dissatisfaction and Complaining Behavior, 17, 27–41.
Söderlund, M. & Rosengren, S. (2008). Revisiting the smiling service worker and customer satisfaction. International Journal of Service Industry Management, 19(5), 552–574. https://doi.org/10.1108/09564230810903460
Voutilainen, L., Henttonen, P., Kahri, M., Kivioja, M., Ravaja, N., Sams, M., & Peräkylä, A., 2014. Affective stance, ambivalence, and psychophysiological responses during conversational storytelling. Journal of Pragmatics, 68, 1–24. https://doi.org/10.1016/j.pragma.2014.04.006
Appendix 1. Transcription symbols
. | Falling intonation |
, | Level or slightly rising intonation |
? | Rising intonation |
↑ | Upward intonation pattern |
↓ | Downward intonation pattern |
: | Sound stretch |
really | Stressed syllable |
(.) | Pause, less than 0.3 seconds |
(0.5) | Length of pause |
[ ] | Overlap |
((laughs)) | Transcriber’s descriptions or comments, contextual information |
(–) | Indecipherable |