This article examines how AI can help with the fight against climate change, and particularly in assessing the carbon footprint. Additionally, we are reflecting the development of AI solutions in the company as a part of business reform, especially from the perspective of SMEs.
As an example, we use the SME Snowfox.AI, where these perspectives become concrete. This article also utilizes the knowledge and experience of the AI-TIE project, which explores the role of artificial intelligence and spars SMEs in the introduction of artificial intelligence, especially in the clean industry and health technology sectors.
Carbon footprint and emission reporting
The carbon footprint appears in the companies’ business processes, and almost every product, service or function has positive and negative climate impacts. From a business perspective, calculating the carbon footprint is a complex challenge, as up to 90 per cent of emissions can arise from operations outside the company. Traditionally, emissions are reported in corporate responsibility reports on annual basis, focusing on fuels and mileage as well as electricity and heat consumption, for example. Comprehensive calculations at the level of business processes, on the other hand, are difficult to make.
Globally, reducing emissions is an important climate objective. The EU Climate Act requires carbon neutrality by 2050. Finland’s goal is to be carbon neutral by 2035. These goals have also transferred to the level of companies, part of their everyday lives, and business processes are viewed with a magnifying glass from the perspective of the implementation of eco-principles.
Reporting emissions can even be mandatory depending on the size of the company, industry, and home country. Currently, in around 40 countries, annual reporting of greenhouse gas emissions is mandatory for organizations according to certain criteria (UL Solutions, 2020). For example, the SECR Act, which came into force in the UK in 2019, requires all major companies (approx. 12,000) to report their annual carbon footprint and energy consumption. Similarly, the EU already has a CSR reporting system in place, which will expand in the coming years to cover around 49,000 companies (Abnett & Jessop, 2021). It can be assumed that as the emission targets tighten, the reporting of the carbon footprint is becoming commonplace for an increasing number of companies in Finland as well.
The role of artificial intelligence in the prevention of the climate change
Artificial intelligence can help in the climate crisis in many ways, related in one way or another to process optimization, automation, and forecasting.
With the help of optimization, the use of resources and energy can be reduced, e.g., reducing logistics fuel demand, designing less resource-intensive components, designing more energy-efficient buildings, anticipating, and enhancing electricity generation and monitoring forests and fields (Rolnick et al., 2019). Forecast models can be used to examine how decisions and choices would affect future emissions, allowing the strategy to be changed proactively.
In this context, it should be noted that the artificial intelligence is a major producer of emissions, as the teaching of artificial intelligence models can be energy-intensive. This is especially true to the most advanced deep neural network models. According to one estimate, teaching one such model can produce the same amount of carbon dioxide as a five-car internal combustion engine throughout its life cycle (Strubell et al., 2019). Using a ready-made, already taught model, on the other hand, does not consume significant energy.
The direct and indirect emissions of a company, and their connection to financial management
According to the international GHG protocol, a company’s total carbon footprint can be divided into three different sources: direct own emissions, emissions from the production of energy used, and indirect emissions from supply and production chains (IPCC, 2018). It is estimated that in the US and China, for example, more than 80 % of the emissions come from indirect sources. The estimation of the indirect is very difficult, and the carbon footprints reported by companies are mostly over-optimistic, i.e., smaller than in reality. In the IT sector, for example, actual emissions can be even twice as high as reported (Klaaßen & Stoll, 2021).
Specialized tools and services are available for calculating your carbon footprint, such as Turbo Carbon, Carbon Footprint and Carbon Chain. However, their activities are typically based on question sets and tabulated estimates, or they are limited to certain sectors of the manufacturing industry. On the other hand, all companies have invoicing as part of financial management, which contains information about the company’s purchases and sales. Invoicing is like the bloodstream of a company because it focuses on the company’s activities.
Since a company’s invoicing and carbon footprint are interrelated, it makes sense to combine these two into one tool. Calculating the carbon footprint based on billing data and the emissions database allows the company’s carbon footprint to be estimated at the level of entire business processes with existing data.
The following paragraph is about how this can be accomplished using the model developed by Snowfox.AI as an example. At the same time this case study serves as an example of how a completely new business can be built based on an artificial intelligence solution and how a typical artificial intelligence solution works technically.
Finnish know-how: Snowfox.ai and calculating carbon footprint
The Finnish company Snowfox.AI has developed an artificial intelligence solution that can sort and analyze invoices and calculate the company’s carbon footprint (Carbonfox) based on them. Carbonfox produces real-time information on the carbon footprint of the company’s acquisitions directly from the data. Often companies already have their own applications, so new AI solutions should be designed in a way that allows them to be connected to an existing system without switching to a completely new one. This is also the case with Carbonfox, which can be integrated into existing financial processing.
The AI solution often requires a little more computing capacity than traditional software. In this case, it may be more useful to use an external cloud service with capacity that scales according to demand and is maintained by the service provider. This way the company does not have to worry about the infrastructure itself, which, especially for AI solutions, can differ significantly from basic online services (e.g. websites and e-mail). The most popular service providers in this field include Amazon Web Services, Google Cloud, and Microsoft Azure. Not only the traditional IT infrastructure but also various AI solutions can be built on top of these. In addition to the previous ones, there are a huge number of smaller AI-focused cloud services. Snowfox.AI’s service runs on the Google Cloud platform.
In the accounting automation service, Snowfox.AI receives the customer’s unsubscribed purchase invoices in the available format and makes accounting and routing forecasts for the invoices. An overview of Snowfox.AI’s handling of invoices is shown in Figure 1. As is typical, artificial intelligence is only one, often quite of a small part of a larger process diagram.
Figure 1. AI solution as part of financial management. Overview of the process and rotation of invoices.
As shown in Figure 1, the invoicing company sends the invoice, and the invoicing operator forwards it to the purchase invoicing system. When a purchase invoice arrives in the purchase invoice system, invoices that would otherwise be processed manually are transferred to AI for accounting and routing. The AI predicts accounting and routing for the invoice and returns the invoice back to the purchase invoice system with forecast. At the same time, the teaching of artificial intelligence is taking place, supported by Snowfox.AI’s 24/7 analytics.
The solution offered by Snowfox.AI utilizes several AI methods to process invoices. Computer vision extracts numerical and verbal data from invoices. The latter is processed using the NLP solution, the Natural Language Processing system (Natural Language Processing). This creates structured data consisting of both numerical and verbal data. AI models analyze the data and make predictions about correct accounting and routing. This process is illustrated in Figure 2.
Figure 2. Artificial intelligence as part of invoice processing. The AI solution usually contains many separate components.
Data is the most important aspect of an AI solution, because without data, no AI model can be developed. Once developed, the model’s mission is to process new, similar data and make predictions. In the case of Snowfox.AI, they utilize the company’s historical invoice data and external statistical data, which is needed in assessing the carbon footprint. They can be used to teach the AI model the operating principles by which the carbon footprint can be made visible in the daily invoice stream. Based on these large amounts of data, the company can monitor its carbon footprint in real time and influence practices.
The operation of the model and the forecasts it provides can be viewed with different visual views. This is illustrated in Figure 3.
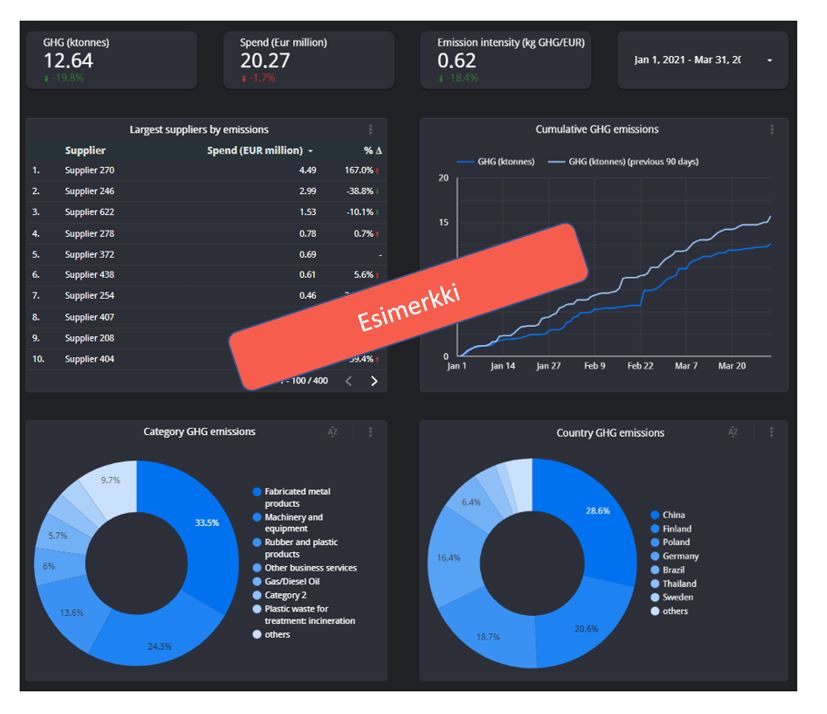
Figure 3. Examples of the customer’s view of the forecasts and results produced by the AI application. Transparency of results and clear visualization are important features for the final user.
The customer must have access to historical purchase invoice data at the outset, either from their own data or from the purchase invoice system vendor because the learning of AI is based on historical data. Snowfox.AI’s solution extracts the information needed for accounting from scanned and e-invoices, such as cost center, VAT code, invoice inspector information, project codes, and customer information.
B2B service productized from an internal development project
New artificial intelligence solutions usually arise from the need to streamline an existing business or product or internal company process. Snowfox.AI’s artificial intelligence solution was driven by an internal development need.
About three years ago, Snowfox’s parent company, Bravedo, ran thousands of invoices that took a long time to process and account for. An internal project produced a solution to this problem and found out that it also had commercial potential. This formed a new business model, which gave rise to a completely new company based on the use of artificial intelligence.
Artificial intelligence models are sometimes very complex, and their application can be challenging for the non-expert. Bringing an artificial intelligence solution into production and to the customer requires investment. It is also important that the added value of an artificial intelligence service can be easily calculated, so that the transition from a traditional solution to an artificial intelligence solution is sufficiently attractive.
Snowfox.AI’s services aimed to be both efficient and effortless. The customer pays the commissioning fee, after which only the processed invoices are invoiced, i.e., when the savings accrue. Pricing is therefore value-based. The payback period for the commissioning investment is relatively short. Learning the process requires only a small investment of time because the solution is attached to the customer’s own software solutions. Time is saved when there is no need to learn new software and user interface. An example of value-based pricing is a situation where an employee’s invoice processing costs 10 euros, while an artificial intelligence solution handles it at a fraction of the cost.
The development of an artificial intelligence model does not usually end with the first version, but the model must be re-taught from time to time as new data accumulates. User feedback can also be used to teach the model. Snowfox.AI’s artificial intelligence learns from the mistakes made by the model, so the accuracy and speed of the model will improve in use.
Business culture as a key enabler of innovation and service in SMEs
Snowfox is a small agile company whose company culture is described by the motto “it’s nice to work together with us”. This is important because people are a key asset for every company and an enabler of innovation. After finding its own compact niche and developing a business model that suits it, Snowfox.AI has focused on utilizing it.
In their work, the staff is guided by a concrete shared vision: mysticism away from artificial intelligence and Finnish expertise into the global market. Removing “mysticism” is generally important so that even an ordinary, non-technical worker does not find artificial intelligence and learning new technology frightening. This is also one of the key results of Haaga-Helia’s Artificial Intelligence is coming – support, know-how and cooperation in order, i.e. in the TT-TOY project, which studied the role of artificial intelligence in expert work.
The “Nice to do together” motto and the “mysticism away from artificial intelligence” vision are also reflected in the customer interface. The threshold for starting to apply artificial intelligence is being lowered in many ways so that companies can quickly capture its benefits. Even in the first consultation, it is emphasized that artificial intelligence is just a learning machine that is good at repeating the same task i.e. accounting invoices. This frees up the potential of experts for more challenging tasks. The free start-up trial starts with a chart of accounts familiar to the customer and gives concrete expression to the possibilities offered by artificial intelligence. It also produces the first estimate of the level of automation and a confidence assessment. Transparency is an important value in the service process: both the AI analysis process and the data generated can be easily interpreted by the customer.
Conclusions
- Carbon neutrality and achieving it is a big goal, and companies can offer small, efficient, targeted solutions. When these factors are combined, significant impact builds up. Snowfox’s AI-based Carbonfox service is a good example of this.
- The AI solution to be productized can start from the company’s internal development needs, in which case the solution will initially be developed and tested in your own company. Once the solution is ready and productized, it will be offered more widely.
- From the SME’s point of view, an AI solution that is targeted at a specific business challenge is functional and cost-effective. One highly competitive product is usually better than many mediocre products.
- Artificial intelligence needs large amounts of data. It is important to find out at the outset where and who owns the data and how the company can get an access to it.
- A powerful AI solution can be one that is built on top of existing processes. The solution can completely replace the previous processes.
- The openness of the company culture to experiments and a good team spirit speeds up the finding of solutions, strengthens the company’s innovation activities and is reflected in the customer and partner interface.
The AI-TIE – Artificial Intelligence Ecosystem Competitiveness for SMEs project supports SMEs in developing and growing their business by utilizing AI solutions to improve internal business processes and innovation work in the product and service development phase and in the sale and delivery of products and services to customers. The project is supported by the European Regional Development Fund and the Uudenmaan liitto. The project is funded as part of the European Union’s response to the covid-19 pandemic.
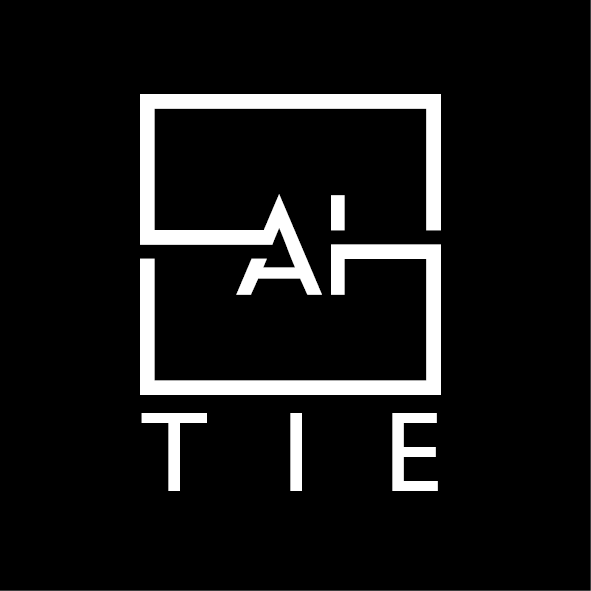
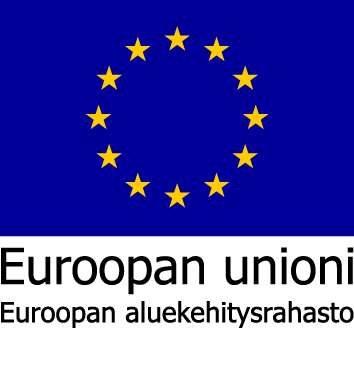
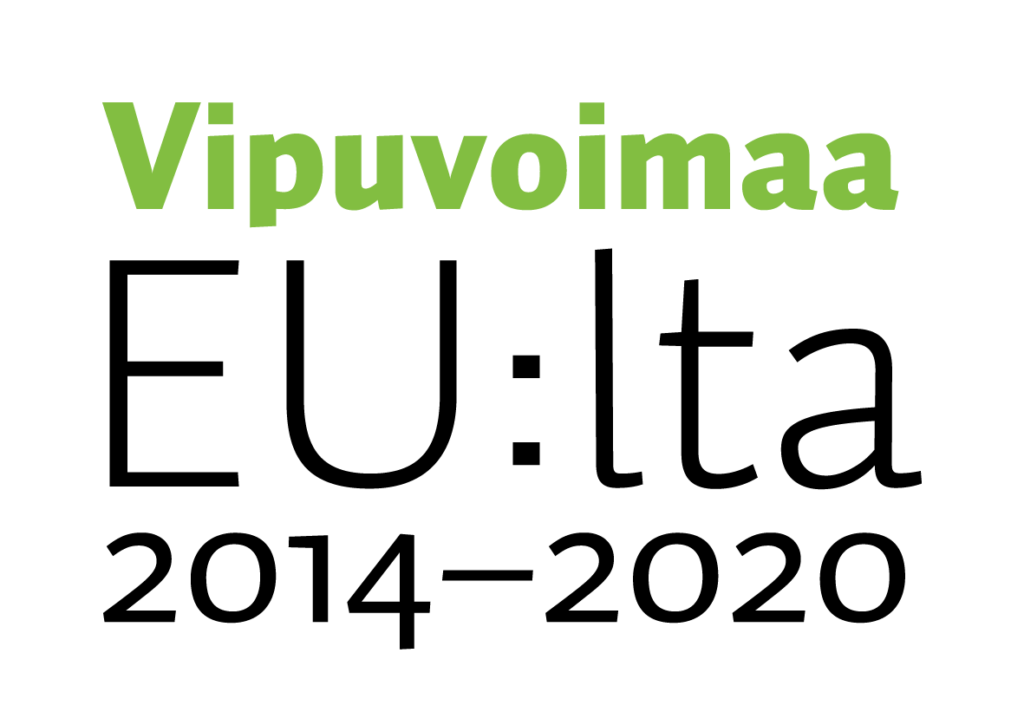
Translated from the Finnish by Sari Benford and Petteri Saloranta.
Sources
Abnett, K. & Jessop, S. 2021. EU broadens rules on sustainability data to capture more companies. Reuters. Accessed: 6 March 2022.
IPCC 2018. Special report: Global Warming of 1.5 ºC. Accessed: 6 March 2022.
Klaaßen, L. & Stoll, C. 2021. Harmonizing corporate carbon footprints. Nature communications, 12, 1, 6149.
Rolnick, D., Donti, P. L., Kaack, L. H., Kochanski, K., Lacoste, A., Sankaran, K., & Bengio, Y. 2019. Tackling climate change with machine learning. arXiv preprint, arXiv:1906.05433.
Strubell, E., Ganesh, A., & McCallum, A. 2019. Energy and policy considerations for deep learning in NLP. Proceedings of the 57th Annual Meeting of the Association for Computational Linguistics, pp. 3645–3650.
UL Solutions 2020. Mandatory Emissions Reporting Around the Globe. Accessed: 6.3.2022.