Today’s business landscape recognizes the strategic importance of leveraging knowledge assets as a primary source of competitive advantage. The importance of consolidating the wealth of knowledge in a manner that preserves and makes it easily accessible has been acknowledged for decades, leading to investments in knowledge management since the 1990s. However, these efforts traditionally demanded significant labor and were not very effective. Company personnel still spend large amounts of time consolidating information from various fragmented sources such as document management systems, databases, note-taking tools, internal data repositories, document sharing systems, emails, and so on. According to studies conducted by IDC (Probstein 2019), employees and decision-makers still spend too much time gathering information instead of analyzing it.
Opportunities of using generative AI for knowledge management processes
ChatGPT, as the main example of generative AI, demonstrated fantastic opportunities for working with humankind’s knowledge base – you can ask how to fix any Windows 10 problem, learn how to write Python code or cook the cake you tried yesterday. Similar opportunities are becoming available for companies to leverage organizational knowledge assets – imagine ChatGPT that gives answers using your corporate documents and data. Generative AI is revolutionizing knowledge management by automating the capture and update of knowledge bases, facilitating rapid content creation, enhancing search capabilities, identifying knowledge gaps and duplicates, and streamlining governance processes (Murphy 2023). Forbes highlights how these AI advancements are fundamentally changing the landscape of knowledge management, making it more efficient and effective (Eliyahu 2023). Shelf.io underscores AI’s role in connecting information from diverse sources, emphasizing the technology’s capacity to unify disparate data, thereby offering a more holistic view of knowledge (Shelf 2024). The role of generative AI in the knowledge management processes (creation, storage and retrieval, transfer, application) at a more conceptual level was analysed and reflected by “classics” of knowledge management systems (Alavi et. al. 2024).
Some companies already implemented AI to leverage their knowledge assets. McKinsey recently introduced Lilli, their new generative AI tool: “Lilli aggregates our knowledge and capabilities in one place for the first time and will allow us to spend more time with clients activating those insights and recommendations and maximizing the value we can create,” says Erik Roth, a senior partner with McKinsey who leads the firm’s development of Lilli and integrating generative AI technologies into the company’s work (McKinsey 2023).
The recent advances in generative AI technology, especially in Large Language Models (LLMs), enable the exploration of vast amounts of unstructured and structured data through convenient conversational interfaces. Generative AI can easily provide chatbots for internal know-how (Wired 2023), utilizing a company’s vast but scattered intellectual capital. Large language models (LLMs) streamline knowledge access by applying their advanced language processing and reasoning capabilities to corporate documents. A user can get answers to such questions as “What steps to do to prepare a new project?” or “What are the success factors of launching a new digital service?”. A multitude of AI-driven tools are readily available and can be assembled into company-tailored solutions for creating, organizing, and finding information (Khan 2023).
Also, access to human capital & expertise of people can be transformed using AI. Generative AI can identify employees’ skills to match them with business needs. For example, answering such questions as “Who is an expert in topic X?” or “What are the key competencies of our department?” is much easier with contemporary AI tools (Kudryavtsev 2024).
To make generative AI useful for companies, LLMs need to be tailored to the needs of a specific organization. This can be done via training or fine-tuning LLMs using company-specific data, but it is a resource-intensive and expensive process, especially for small and medium-sized companies. Therefore, the prevalent approach is to input relevant information to existing LLMs using so-called “retrieval-augmented generation” (RAG) technology (Amazon 2024).
The need for knowledge models to address the limitations of generative AI
However, generative AI still struggle with several risks and challenges: hallucinations (where the AI system generates false or misleading information), lack of provenance and predictability (tracing the origin and creation process of the output produced), lack of context (correct understanding of users information need), lack of timeliness (risks related to freshness and up-to-date data and response) (Deloitte 2023). Queries pertaining to routine business operations could be complex and may require information retrieval from multiple documents. For example, a new project manager at a software development company may have a question like “Who are the key experts in TechCorp’s cloud computing division, and what were their roles in the last major project?”
Domain-specific knowledge models such as knowledge graphs can be used to address the knowledge access limitations of LLMs (Deloitte 2023). These knowledge models define concepts and relationships for the selected domain. This approach leverages data preparation, identifies relationships within the data, and understands the query context. The primary objective of this approach is to transform the way organizations leverage their data assets. It is particularly relevant for medium-sized companies that often have large volumes of both structured and unstructured data but limited expertise and resources.
This transformation focuses on enabling companies to increase the quality of LLM answers and generate insights, tailored to their unique business needs. This innovation enables the employees to use domain-specific assistants/copilots to converse with the companies’ information to get precise and relevant answers to their questions. Internal data can be easily integrated with external sources of information. As a result, it leads to enhanced productivity, and informed decision-making, saving experts’ time and sparing them to focus on value-adding tasks, better work quality, and better work satisfaction.
Example application areas for knowledge-enhanced generative AI for enterprises
Generative AI is now penetrating many areas (Chui et al. 2023). In the following, we list potential application areas of knowledge-enhanced generative AI in business.
Customer Support: Knowledge-enhanced generative AI can improve the quality and efficiency of customer support services. For instance, such a system can provide immediate and personalized responses to complex customer inquiries regardless of the language or location of the customer (Chui et al. 2023). It can provide detailed product specifications, usage instructions, or pricing information, significantly reducing customer wait times. This system can provide technical assistance by guiding users through troubleshooting steps. For instance, if a customer has an issue with a software product, the AI system can understand the problem’s context and offer a step-by-step solution, pulling from the data of previous similar cases and their resolutions.
Sales Enhancement: In sales, AI-powered solutions help to improve performance and productivity (Salesforce 2024). Knowledge-enhanced generative AI brings a new level of sophistication to sales by recommending personalized products or services and handling routine sales inquiries. The system achieves this by understanding the semantic relationships between items, which means it recognizes the connection and similarities between different products or services based on various factors like features, usage, user ratings, etc. Regarding routine inquiries, if a customer inquires about the availability of a new organic skincare product, delivery options, or ongoing promotions, the AI system can promptly and accurately respond. This enhances customers’ shopping experience and potentially improves sales outcomes.
Service Design and Improvement: The AI-driven solutions can also significantly enhance the process of service design and improvement. They can interpret and analyze customer feedback, identifying pains and needs that might not be immediately apparent. Moreover, the AI system can generate recommendations by combining insights from various data sources. For instance, it might suggest elements of value proposition for the restaurant based on industry-specific research reports and articles about success factors and service quality.
Business Development: AI-driven solutions can identify potential market gaps or consumer trends from various data sources like market reports, social media sentiment, and competitor activities. These insights can help a business tailor its offerings and position itself effectively as it enters a new market. Furthermore, the AI system can support the ideation process during business model design using both market analysis and existing business model patterns.
Employee Onboarding Process: An AI-driven system can serve as an interactive resource for new hires, answering questions about company policies, procedures, and culture. For instance, suppose a new employee inquires about his business travel plan. This question could encompass multiple areas, such as the company’s stance on booking travel, travel expenses reimbursement, per diem allowances, and the process for claiming these expenses. Answering this question would require accessing and synthesizing information from several resources. Furthermore, the system can customize the onboarding/training content for the specific role or position of a new employee – needed knowledge for a new researcher is different from the one for a project manager.
Skills Management & Expert Search: The AI-driven knowledge access system can pinpoint areas where the workforce may lack certain skills, thereby helping to focus on necessary training and development initiatives. For instance, if the system identifies a gap in digital marketing skills across the organization, it can suggest implementing a company-wide training program on this topic. Similarly, the system can find the right experts for specific projects. Suppose a project requires expertise in data analysis. The AI system, by analyzing employee profiles and skills data, can identify individuals with strong data analysis skills. Moreover, the system can provide specific upskilling recommendations. For example, if it identifies a recurring need for blockchain expertise in upcoming projects but finds a lack of this skill within the organization, it can suggest relevant training or certification programs.
The suggested approach for knowledge-enhanced generative AI for business is summarized in Picture 1.
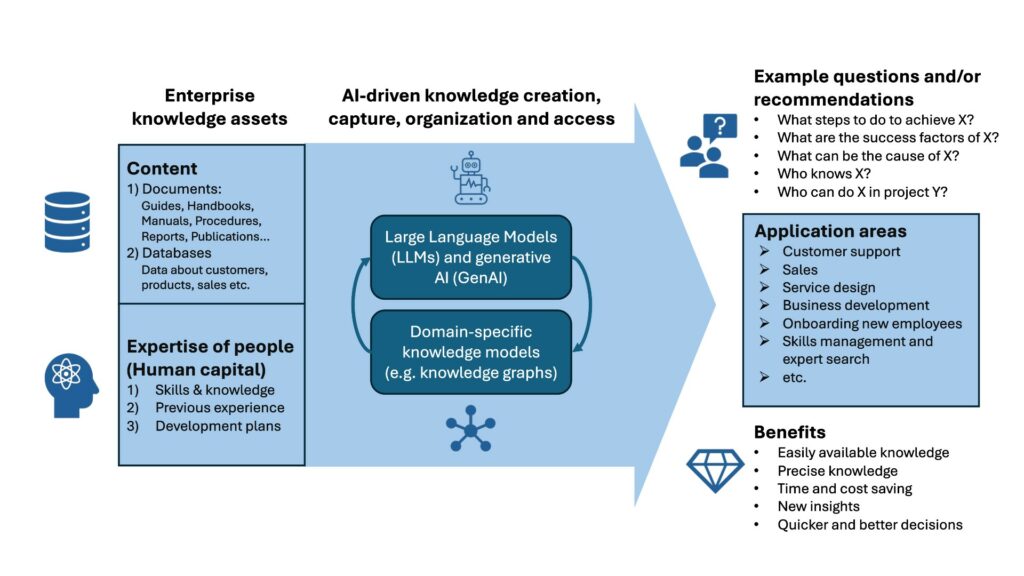
Picture 1. Overview of the knowledge-enhanced generative AI for business (created by authors)
Making knowledge-enhanced generative AI Work
There is vast potential to improve knowledge access via generative AI and LLMs. Existing challenges and limitations of LLMs can be addressed by domain-specific knowledge models such as knowledge graphs. The building blocks for creating next-generation knowledge access systems are suggested within the research and independent prototypes. To make these approaches work in business, the technologies should be customized to the needs of specific application areas. Industry-university cooperation can help to accelerate this process.
In response to the growing need for AI-driven knowledge access in businesses, we initiated a research and development project with academic and industrial partners. The project aims to craft a research challenge using a co-creation approach and subsequently co-develop multiple prototypes of 2-3 relevant application areas. We have started the process of establishing collaboration with industrial and academic partners. Using a co-development approach in collaboration with industrial partners, serving both as solution users and/or technology providers, we aim to provide businesses with precise access to their knowledge.
References
Alavi, M., Leidner, D. E., & Mousavi, R. 2024. A Knowledge Management Perspective of Generative Artificial Intelligence. Journal of the Association for Information Systems, 25(1), 1-12.
Amazon. 2024. What is RAG. Accessed: 13 March 2024.
Chui M., Hazan E., Roberts R., Singla A., Smaje K., Sukharevsky A., Yee L., Zemmel R. 2023. The economic potential of generative AI: The next productivity frontier. McKinsey & Company. Accessed: 13 March 2024.
Mckinsey & Company. 2023. Meet Lilli, our generative AI tool that’s a researcher, a time saver, and an inspiration. Accessed: 13 March 2024.
Deloitte. 2023. Responsible Enterprise Decisions with Knowledge-enriched Generative AI. Accessed: 13 March 2024.
Eliyahu S. 2023. How Generative AI Is Revolutionizing Knowledge Management. Forbes. Accessed: 13 March 2024.
Khan U. 2023. The Unstoppable March of Artificial Intelligence: The Dawn of Large Language Models. eSignalsPRO. Haaga-Helia. Accessed: 13 March 2024.
Kudryavtsev D. 2024. Bridging knowledge gaps: AI-enhanced techniques for identifying skills and competencies. eSignalsPRO. Haaga-Helia. Accessed: 13 March 2024.
Probstein S. 2019. Reality Check: Still Spending More Time Gathering Instead of Analyzing. Forbes. Accessed: 13 March 2024.
Murphy T. 2023. How generative AI can improve knowledge management. TechTarget. Accessed: 13 March 2024.
Salesforce. 2024. Sales AI. Accessed: 13 March 2024.
Shelf. 2024. The Role of Artificial Intelligence in Knowledge Management. Accessed: 13 March 2024.
Wired. 2023. Three tech micro-trends businesses need to know. Accessed: 13 March 2024.
Picture: Shutterstock