Problem description and motivation
In the rapidly evolving business environment, recognizing and comprehending skills, knowledge, and competencies at multiple levels — from individuals to teams, departments, and the organization as a whole — is crucial. This understanding is particularly vital for executives, heads of departments, project coordinators, and team leaders. It is about addressing fundamental questions: What expertise do we possess at each level (e.g. in a team, department, organization), and who are the key knowledge holders? At the same time understanding the required skills, knowledge, and competencies is equally important: what do we need to know to achieve our goals, implement strategy, provide specific business capabilities, respond to market demand, perform business processes, and do projects and jobs? Being aware of knowledge supply and demand is essential in the knowledge economy.
This understanding is vital for different stakeholders of an organization:
- Managers want to know the core competencies of the organization/unit to develop strategy and development plans. They also need to understand the competencies of employees to plan new projects and establish teams, as well as knowledge and skill gaps for further development,
- Employees benefit from knowing their colleagues’ areas of expertise to seek advice or answers. They also need to understand the required skills for personal career planning and development,
- Potential partners look to see if your business has the necessary expertise in specific fields before deciding to collaborate,
- Customers, especially in knowledge-intensive service industries, would like to know the knowledge capabilities of an organization when they select product or service providers for long-term relationships.
These interests can be found in different types of organizations, public and commercial, in different industries. For example, it also applies to universities and organizations in academia. The administration is interested in whether the university has the necessary competencies to launch a new educational program or research project. Research staff are interested in potential collaborators for a new/potential research project. Educational program managers look for lecturers for specific courses or guest lectures. Students search for experienced supervisors for their thesis. Potential partners search for knowledgeable consortium members for grant applications.
Existing approaches for skills and knowledge identification
Identification of people’s expertise in a business context is addressed by methods and tools from the following disciplines: knowledge mapping, expertise location and skills management. Digitalization made these methods and tools data-driven, while recent advances in AI allow us to automate many steps without high technical skills.
The term Knowledge map was used with various meanings in several scientific communities: knowledge management, education studies, organization studies, decision analysis, artificial intelligence, etc. We will use the meaning from the knowledge management area. According to APQC (2022), a knowledge map visually represents an organization’s knowledge resources. A knowledge map acts as a “snapshot in time” to help an organization understand what knowledge is critical to a business process or focus area, where that knowledge resides, how knowledge flows between people and systems in the course of work, and any gaps in knowledge or issues that impede its movement. A recent survey (APQC 2020) defined knowledge mapping/identification of critical knowledge as the most influential technique to help knowledge management respond to current changes and meet evolving needs.
One of the foundational articles identified the following knowledge map types (Eppler 2004).
- Knowledge source maps (where the knowledge is)
- Knowledge asset maps (what kind of knowledge we have)
- Knowledge structure maps (how the knowledge is organized and interconnected)
- Knowledge application maps (which knowledge is needed for performing activities, producing required results, and achieving goals)
- Knowledge development maps (how certain knowledge can be developed, e.g. via courses)
A systemic overview of knowledge mapping was done in Balaid et al (2016), while the typology and knowledge maps’ structure were studied in Kudryavtsev et al (2022). Knowledge mapping methods and tools address the questions from the first section, but they are also broader since they cover both the knowledge of employees and documented knowledge.
“Expertise location or expert finding has long been a valuable approach for very large and globally dispersed organizations. With the increase in remote and hybrid work as well as the current talent shortage, expertise location has become critical for mid-size and even small companies.” (APQC 2021.) The first expert-finding systems appeared in the late 90s and early 2000s (Yimam-Seid & Kobsa 2003). The works in this area paid a lot of attention to identifying sources/indicators of expertise. They suggested various algorithms and technologies for locating and recommending experts for a specific topic. A recent overview of the field can be found in Husain et al. (2019) and APQC (2021). These methods mainly help to identify employees’ existing knowledge.
Skills and competency management is also a mature topic in human resource management (Bersin 2023). Skills are the central element of HR processes: recruitment, assessment, training, and career planning. Skills or competency models are created in this discipline, both universal (e.g. ESCO is the multilingual classification of European Skills, Competences, Qualifications and Occupations) and domain-specific (e-CF – EN 16234-1 e-Competence Framework). Skills management is especially “hot” now since AI has given additional capabilities for skills identification, assessment, categorization, etc., which resulted in the emergence of SkillsTech (Bersin 2021). These methods and tools help to identify not only existing skills but also required ones, e.g., based on the analysis of vacancies, see (Khaouja et al. 2021) or Skills-OVATE platform, which offers detailed information on the jobs and skills employers demand based on online job advertisements in 28 European countries. Existing and required skills help to define the skill gap and suggest measures for eliminating it (e.g. courses for upskilling).
Identification of existing and required knowledge as a data analysis process
Since the identification of existing and required knowledge has become extremely data-driven nowadays, it can be considered as a data analysis process with the following steps: 1) Data collection, 2) Data processing and analysis, 3) Data visualization and access.
1. Data collection
The following sources can be used to identify existing skills, knowledge and competencies of employees: self-reporting, surveys, peer-to-peer assessment, content authorship (documents, publications, posts, etc.), membership and person’s activity in online groups, tasks and projects performed, courses taught etc.
What data/sources can be used to define the required knowledge? If you think about individuals/employees, then texts of vacancies or job descriptions can be used to extract needed skills and knowledge. If you think about teams or departments then the list of business processes with a necessary decomposition or projects’ work breakdown structure can be used. At the level of organization, the decomposition of strategic objectives and business capabilities, product and service portfolio, and customer segments can generate needed competencies and knowledge.
2. Data processing and analysis
Natural Language processing including Large Language Models (e.g. ChatGPT) can be utilized here.
A computer can extract topics from the texts, which represent existing or required knowledge.
For example, you have 30 documents written by an employee, and you need to extract 10 topics, which describe these documents as well as the employee’s competencies. When analysing knowledge needs you can take a set of vacancies and ask the computer to identify the most cited knowledge and skills. Knowledge, skills or competencies can be organized into taxonomies, skills/competency models or knowledge graphs (Kudryavtsev 2022). These predefined lists can be used for classifying texts, which reflects skills/knowledge. Such analysis may help to assess the “amount” of expertise, for example, how many publications were written on each topic in the taxonomy. Since knowledge mapping can address different levels in an organization (e.g. employee, team, department, whole organization), data integration, merging, aggregation and generalization are usually required. For example, if we define the competencies of individuals in several departments, aggregated representations are needed to analyze and compare the knowledge profiles of these departments. Unified taxonomies are especially useful for such aggregations – they not only provide common vocabulary but also include generalization.
3. Data visualization and access
The results of data analysis – information about existing and required knowledge/skills – should be readily available for decision-makers and employees. Visualization and user interfaces for searching, filtering, asking questions, and browsing are used at this step. Since data about skills, knowledge and competencies is a subtype of data, various data visualization techniques can be used – see The Data Visualisation Catalogue. For example, a bar chart can demonstrate how many experts an organization has in different domains, but if we have a hierarchy of domains then Treemap, Circle packing or Sunburst diagrams can be used. Different types of tools can be taken for the visualization: RAWGraphs – a free and open-source tool for data visualization, data visualization libraries for Python (e.g. Seaborn), commercial interactive data visualization software (Microsoft Power BI, Tableau etc.). No-code databases like Airtable, which combine the simplicity of a spreadsheet with the complexity of a database, such tools provide filtering and customized views.
There are also complex software products that support all these steps, such as CornerStone, Workday, and Skills in Microsoft Viva. However, these products are heavy-weight and are usually a part of integrated talent management platforms. So, if you are starting to identify skills/knowledge, lightweight tools, which can be tailored to the specific use case, are preferred.
Benefits from understanding existing and required knowledge
If knowledge supply and demand are identified (existing and needed), this may generate a multitude of benefits.
- Stronger project teams, where the members complement each other and provide the needed team’s competency profile,
- Strategy and plans will align the company’s knowledge strengths and weaknesses with opportunities and threats (see TOWS matrix),
- Upskilling and training programs will help to address business needs and eliminate justified gaps,
- Partnership and outsourcing will enable organizations to focus on their core competencies and eliminate competency gaps.
Such benefits are nicely demonstrated by tools, which allow a person to enter his/her own skills (or data to extract them, CV, for instance), find the desired job/occupation via AI-based match-making with skill requirements, identify the skill gap between own skills and required ones, and receive a list of available courses to fill the gap. CareerBot, created by 3UAS-universities of applied sciences (Haaga-Helia, Laurea and Metropolia) to help students find jobs, courses, and thesis topics, is one example of such a tool. Another example – the APS Career Pathfinder tool, created by the Australian Government, helps a person to explore potential roles in the Australian Public Service based on his/her skills or interests; then it shows the needed skills to take the selected role and training specific to the skill needs.
Simple first steps to start knowledge identification
Data collection
For business organization: Collect a list of tasks done by employees in the previous year.
For research organization: Collect a list of publications’ titles and abstracts written by your employees.
For educational organization: Collect a list of courses provided by your employees.
Data analysis
Ask ChatGPT to extract topics (for example, 10) from the list of tasks/publications.
Ask ChatGPT to classify a list of tasks/publication titles between 5-10 topics.
Count the number of employees with expertise in each topic area (you can assign different weights to junior, senior and expert level specialists).
Data visualization and access
Create a skills matrix for your team: people are in rows, skills area – columns, level of expertise at the intersection (Picure 1). Expertise levels can be specified using several criteria.
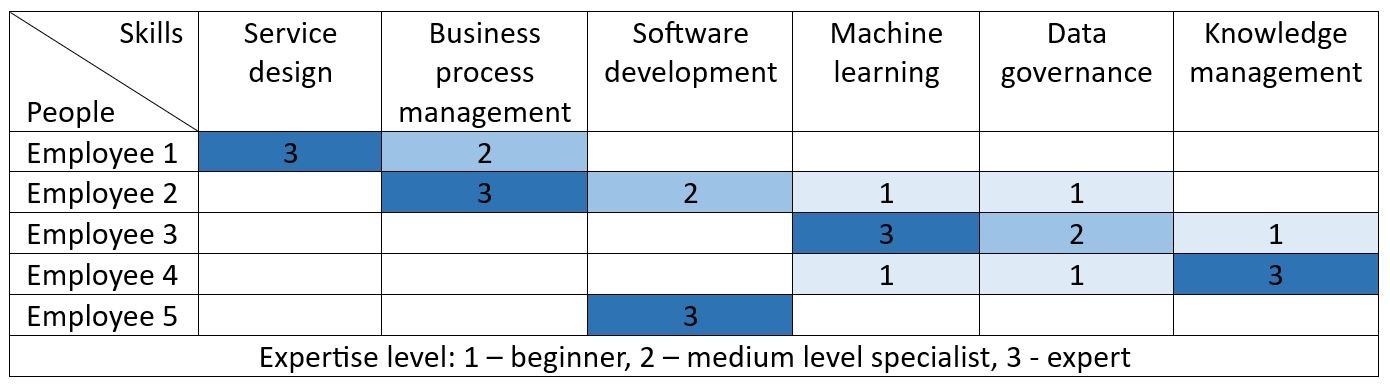
Picture 1. An example of a skills matrix
Think about expertise areas of your company/department/team and create a treemap diagram to represent the “amount” of expertise by topic (Picture 2). It reflects the number of experts in each subtopic using rectangles’ squares (the larger the rectangles, the more experts). This diagram can be created in MS Excel based on the table on the left. Such a diagram can highlight the knowledge capacity of the company in different topics from a certain topic taxonomy.
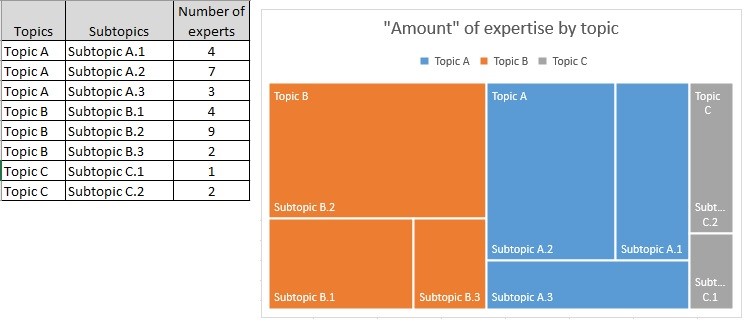
Picture 2. An example of a tree map diagram to represent the “amount” of expertise by topic area
Ethical considerations
Ethics, in a broad sense, refers to the principles of right and wrong that guide an individual in making decisions and conducting their actions. In a business context, ethics pertains to applying these principles to ensure fairness, transparency, and respect for all stakeholders involved.
When identifying employee skills, the following ethical issues should be considered: privacy and consent, data accuracy and representation, and compliance with GDPR. Employees have a right to privacy and control over their personal data. To respect employees’ privacy, in some cases, explicit informed consent can be needed, especially if data for skills identification will be extracted from non-publicly available sources. The data collected must accurately represent the employees’ skills and competencies. Ensuring that the methods and criteria used for data collection are fair, transparent, and objective is essential to maintain trust and effectiveness in the skill identification process. Compliance with GDPR involves ensuring the lawful processing of personal data, safeguarding data security, and upholding the rights of employees as data subjects. Employers must also conduct data processing transparently and limit data collection to what is necessary for the intended purpose.
Contextual factors will influence the importance of the aforementioned issues. The intended skills data usage is relevant. For example, using skills data for performance reviews and rewards requires a more careful and considered approach than using the same data for creating project teams, knowledge sharing and upskilling recommendations. There are also significant regional peculiarities of employee data usage related to ethical concerns, privacy, and consent, for example, for Europe, Asia, Africa and even for specific countries. These differences are often rooted in varying legal frameworks and cultural attitudes towards privacy and employee rights.
So, what is needed to work with skills and knowledge ethically? Transparency is key to addressing these issues. Communicate the purpose, scope, and data collection methods to the employees. Obtain explicit consent where necessary and allow employees to opt-out or control how their data is used. Ensure that data processing methods are fair and objective and keep the data secure to prevent unauthorized access.
Wrap-ups
In conclusion, identifying existing and required knowledge and skills is crucial for various stakeholders within an organization. This identification can be considered as a data analysis process, leveraging modern tools and technologies like AI, large language models, and data visualization software. Simple initial steps, such as using ChatGPT for data analysis and creating skills matrices, enable organizations to start aligning the intellectual capital of their people with strategic goals and business needs. Ethical issues should be considered when identifying, analyzing and sharing information about employees’ skills.
References
APQC. 2022. Getting Started with Knowledge Mapping.
APQC. 2021. Understanding Expertise Location.
Balaid, A., Abd Rozan, M. Z., Hikmi, S. N., & Memon, J. 2016. Knowledge maps: A systematic literature review and directions for future research. International journal of information management, 36(3), 451-475.
Bersin, J. 2021. Understanding SkillsTech, One Of The Biggest Markets In Business.
Bersin J. 2023. Building A Skills-Based Organization: The Exciting But Sober Reality.
Eppler M. 2004. Making knowledge visible through knowledge maps: concepts, elements, cases. In: Holsapple, C., ed..Handbook on knowledge management, 1, 189–205. Springer, Heidelberg.
Husain, O., Salim, N., Alias, R. A., Abdelsalam, S., & Hassan, A. 2019. Expert finding systems: A systematic review. Applied Sciences, 9(20), 4250.
Khaouja, I., Kassou, I., & Ghogho, M. 2021. A survey on skill identification from online job ads. IEEE Access, 9, 118134-118153.
Kudryavtsev, D. 2022. Skills knowledge graph curation: the case of digitalization design domain. SEMANTiCS Conference 2022.
Kudryavtsev, D., Gavrilova, T., Grinberg, E., Kubelskiy, M. Map of the Maps: Conceptualization of the Knowledge Maps. BIR 2022 Workshops and Doctoral Consortium, 21st International Conference on Perspectives in Business Informatics Research (BIR 2022), September 20-23, 2022, Rostock, Germany, pp. 14-23.
Yimam-Seid, D., & Kobsa, A. 2003. Expert-finding systems for organizations: Problem and domain analysis and the DEMOIR approach. Journal of Organizational Computing and Electronic Commerce, 13(1), 1-24.
Editing: Marianne Wegmüller
Picture: Shutterstock